This research theme is targeted at arguably the most widely used general class of data in medicine, whereby devices capture images of the human body to aid diagnosis, understanding of disease pathophysiology and prediction of disease evolution. It is a hallmark modality for clinical decision making. With AI we see a radical shift in how data originating from imaging devices are analysed with notable penetration of AI into the market (e.g. in 2023, 396 of the 520 FDA approved AI medical algorithms were in radiology). Key challenges remain in generalisation, fairness, and multi-modal learning with considerable activity in this field also heavily influenced by foundational models also including vision-language models.
This theme benefits by an ever-growing ecosystem in Edinburgh and Scotland. For example, Edinburgh is home to CHAI – EPSRC AI Hub for Causality in Healthcare AI with Real Data aiming to see how causality can help address questions of generalisation and fairness. There is also large pull from large scale imaging research groupings, for example Edinburgh Imaging is the University’s academic hub and expertise with respect to imaging/analysis/experiments and facilities and SINAPSE is a Scottish wide network of imaging scientists both clinical, technical and academic.
Projects in this theme will aim to address these challenges by devising new theoretically grounded but applicable solutions that can scale to address ever pressing needs in interpreting and understanding medical images.
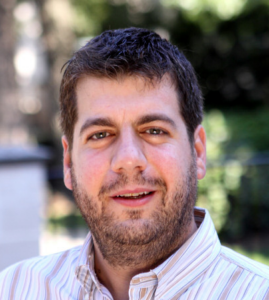
Professor Sotos Tsaftaris
Research Theme Lead
AI for Biomedical Imaging
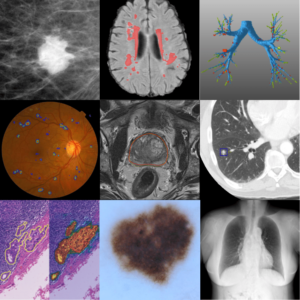